Improving solar storm modeling with machine learning
With the STEREO-A spacecraft passing the L5 point of the Sun-Earth system in 2020, its heliospheric imagers (HI) provide white-light observations of CMEs from their launch at the Sun up to Earth from changing viewpoints until 2027 when it will reach L4. HI data are perfectly suited to be used as input for CME modeling and prediction tools. These tools are often developed based on HI science data arriving with a delay of some days. HI beacon data, available in near real-time, however, has a lower spatial and temporal resolution and is therefore less qualified to form the basis of accurate predictions.
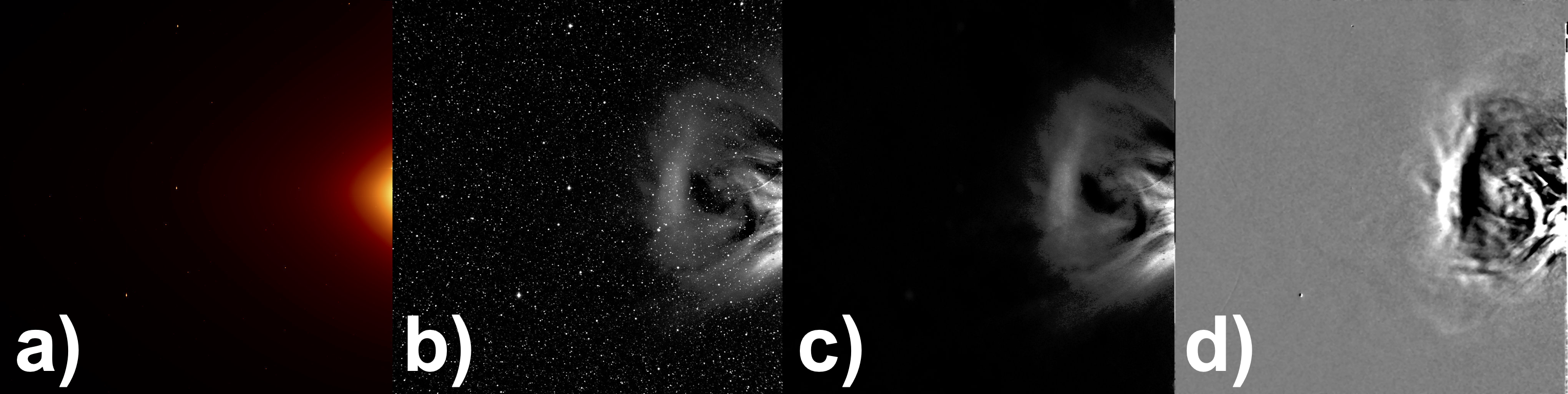
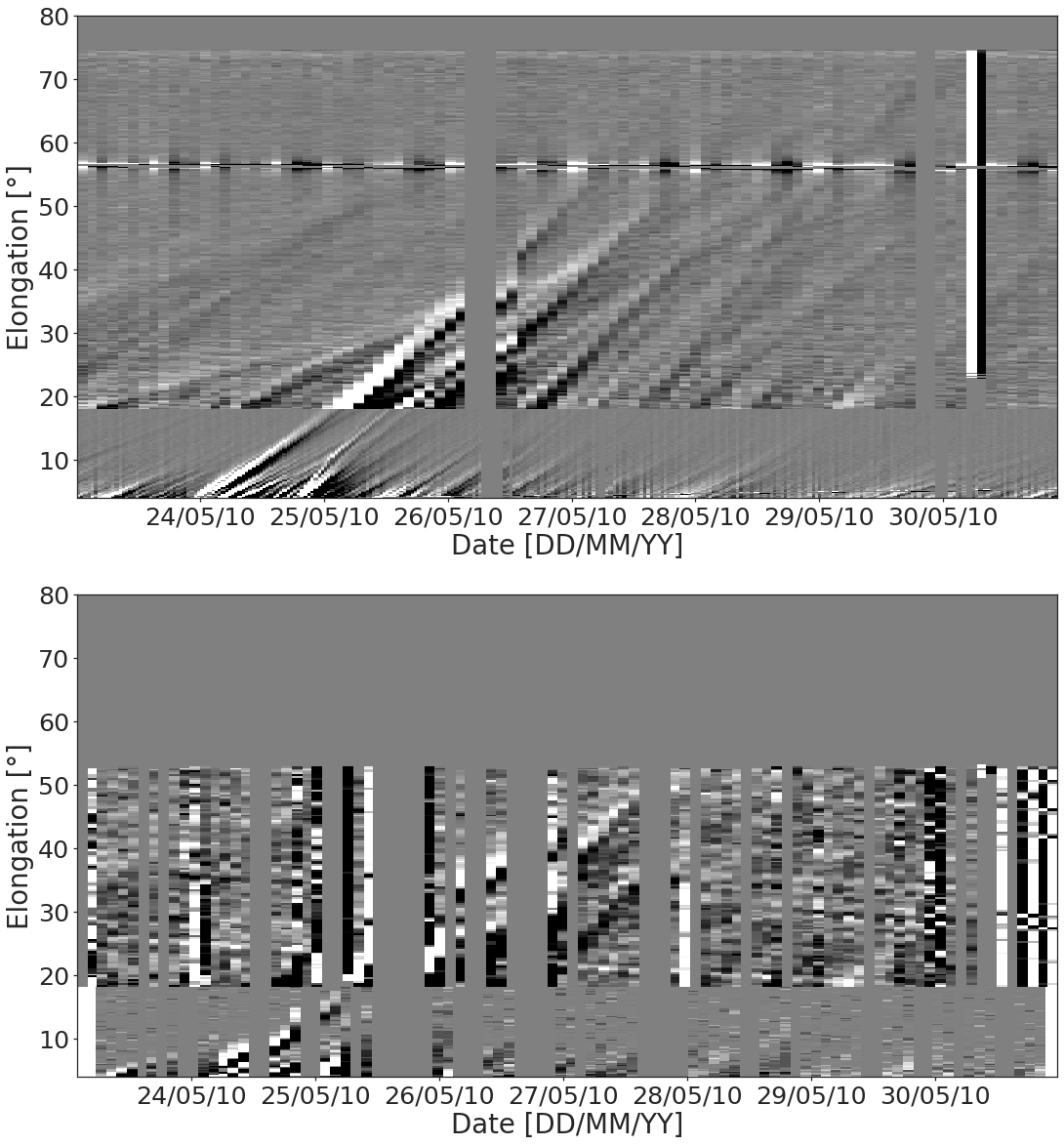
In this project, we aim to enhance STEREO's HI beacon data by applying machine learning tools in order to increase the image quality and
to overcome low temporal resolution and data gaps. The resulting artificial HI science data should then serve as input to an HI-based CME
modeling and prediction tool. In addition to the data quality itself, the extraction of information from these data is challenging.
Usually, the elongation of the CME front within HI data is extracted manually leading to large differences depending on the scientist doing
the tracking and even between tracks measured from the same person. By applying an automated CME detection and tracking ML method we aim to
decrease the prediction error arising from the measurements themselves.
Team
PI: Tanja Amerstorfer
Postdoc: Justin Le Louëdec
PhD student: Maike Bauer
Master's student: N.N.
Duration: 03/2023 – 02/2026
Link to FWF project page: P 36093

Acknowledgements: “This research was funded in whole, or in part, by the Austrian Science Fund (FWF) [P 36093]. For the purpose of open access, the author has applied a CC BY public copyright licence to any Author Accepted Manuscript version arising from this submission."